class: title-slide, left, bottom # KTIS ---- ## **STT powered by Data Science** ### 이광춘 ### 2021-02-05 --- class: inverse, middle # Outline ---- .pull-left[ 경제적인 면에 초점을 두어 .green[**디지털 경제**]의 주요한 동인인 .green[**자동화**]와 .green[**기계학습**]에 대해 살펴봅니다. 개인용 컴퓨터의 보급 이후 만들어진 디지털 유산인 사무 자동화(Office Automation) .warmyellow[**문서**]의 한계와 이를 벗어나려고 하는 다양한 최신 움직임도 짚어봅니다. 디지털 전환의 기본을 이루는 .black[**데이터 과학**]의 핵심적인 내용을 다루고, 다양한 데이터 중 하나인 음성을 텍스트로 변환시킨 후 가치를 창출하는 전반적인 과정을 다뤄보고자 합니다. ] <!--end of left-column--> .pull-right[ .left[ 1\. **[.warmyellow[자동화(Automation)]](#automation)** 2\. [기계학습(Machine Learning)](#machine-learning) 3\. [사무 문서](#case-study-document) 4\. [데이터 과학](#data-science) 5\. [STT](#stt) 6\. [마무리](#goodbye) ] ] <!--end of right-column--> --- name: automation-tax # 세무사 업무 변천과정 <br> .center[ 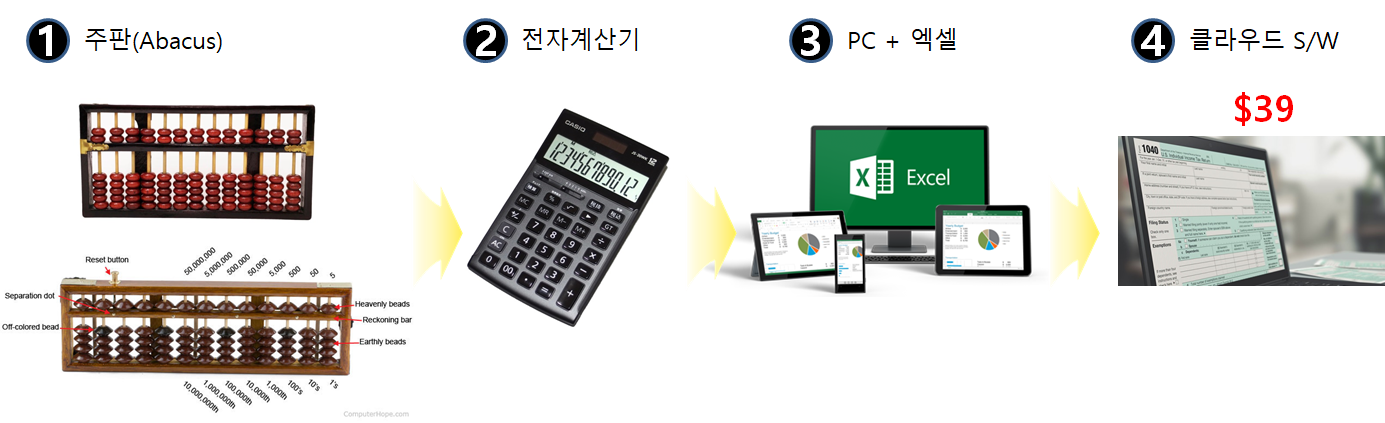 ] .footnote[ [이광춘 (2019-12-04), "자동화(Automation) - ~~로봇~~ 자동화가 빼앗는 일자리 그리고 나", 분당 서현 청소년 수련관 ](https://statkclee.github.io/ds-authoring/ds-bundang-2019.html) ] --- name: automation-productivity-gap # 생산성과 임금 격차
.footnote[ [Economic Policy Institute, "The Productivity - Pay Gap", July 2019](https://www.epi.org/productivity-pay-gap/) ] --- name: automation-sourcing-strategy # 소싱전략의 변화 <br> .center[ 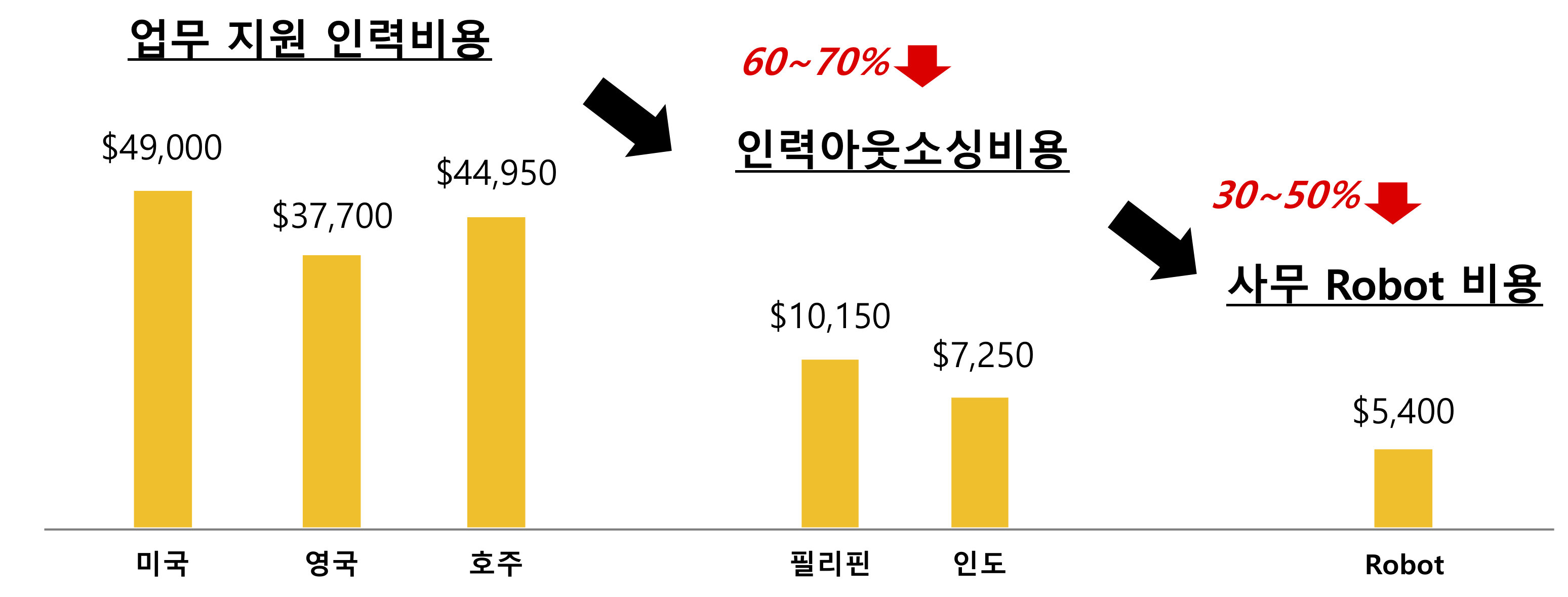 ] .footnote[ 자료출처: Kinetics consulting services / Automation Anywhere ] --- name: automation-paradox # 모라벡의 역설(Moravec’s paradox) <br> .center[ <img src="fig/moravec-paradox.png" width = "87%" /> ] .footnote[ 미국 카네기 멜론 대학 (CMU) 로봇 공학자 한스 모라벡(Hans Moravec)이 1970년대에 ‘it is comparatively easy to make computers exhibit adult level performance on intelligence tests or playing checkers, and difficult or impossible to give them the skills of a one-year-old when it comes to perception and mobility’라는 표현으로 컴퓨터와 인간의 능력 차이를 역설적으로 표현하였다. ] --- name: automation-comparison # 중국어 방 (Chinese room) <br> .pull-left[ 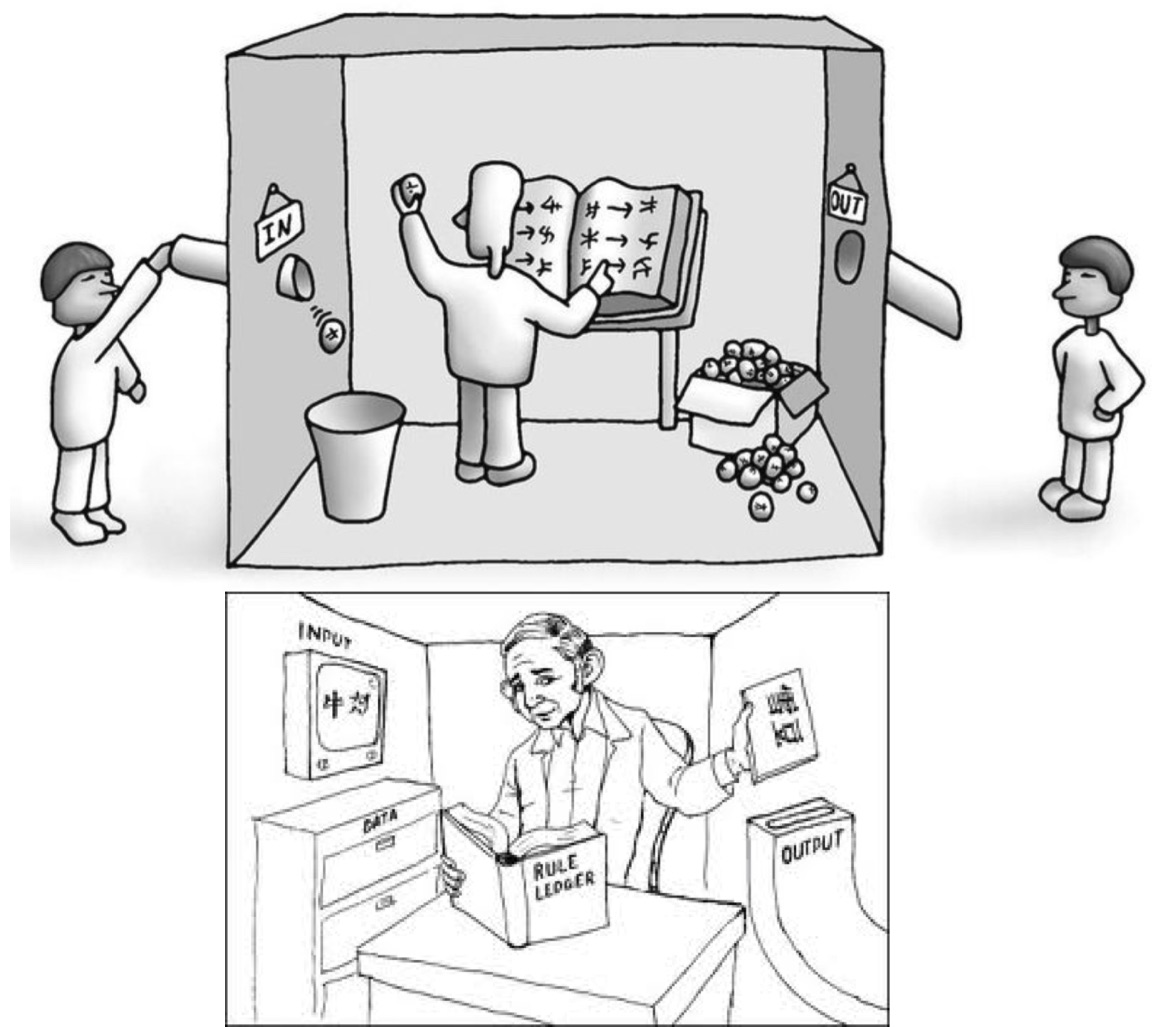 ] .pull-right[ | 인공지능 | 컴퓨터 | 인간 | |:---------:|:-----------------:|:---------------------:| | 중국어 방 | 하드웨어 | 인간의 외형적인 몸체 | | 영어만 할 줄 아는 사람| 소프트웨어| 인간의 지능 | | 중국어로 된 질문 | 입력(Input) | 인간이 외부에서 접할 수 있는 자극 | | 중국어로 된 답변 | 출력(Output) | 인간이 외부에서 접한 자극에 대한 반응 | | 질문&대답 목록 | 데이터베이스(Database) | 습득된 기억 | ] .footnote[ [중국어 방 역설 (Chinese room argument) - 대체 누가 중국어를 이해하고 있는가?](http://ko.experiments.wikidok.net/wp-d/592f718da44f1a4153e80611/View) ] --- name: automation-apollo # 아폴로 우주선 <br> .center[ 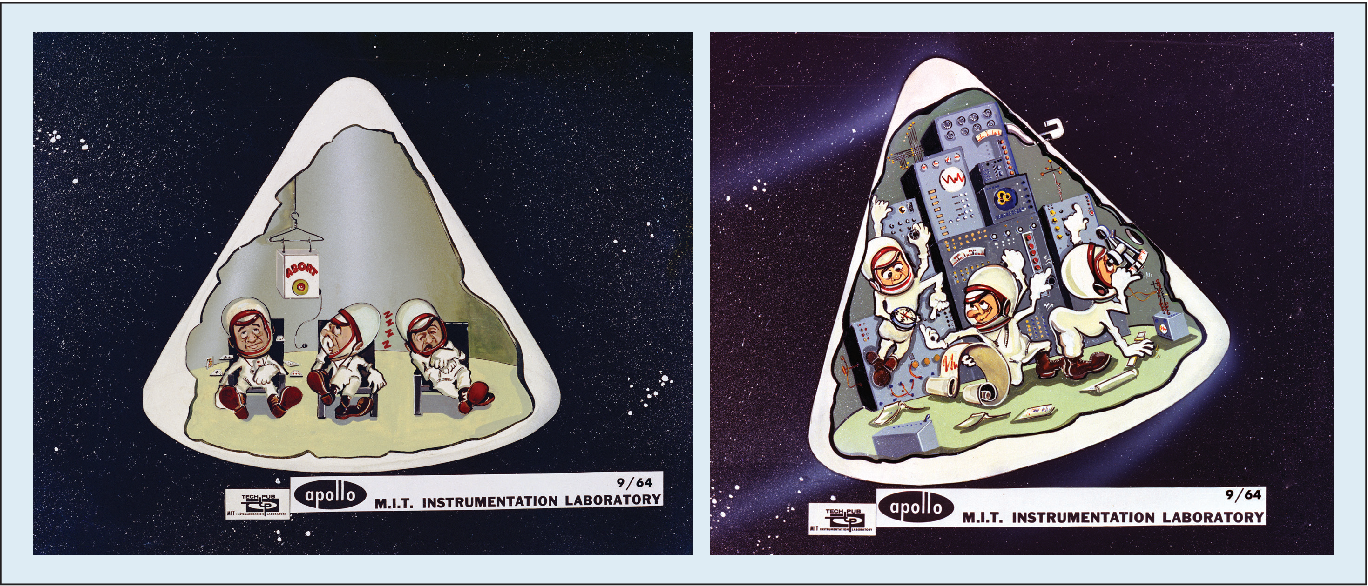 ] .footnote[ [Mary (Missy) Cummings (2014), "Man versus Machine or Man + Machine?", Duke University and MIT](https://hal.pratt.duke.edu/sites/hal.pratt.duke.edu/files/u10/IS-29-05-Expert%20Opinion[1]_0.pdf) ] --- name: automation-role-allocation # 인간과 기계 업무분장 <br> .center[ <img src="fig/role-allocation-skill-rules-experties.gif" width = "77%" /> ] .footnote[ [Mary (Missy) Cummings (2017), "Informing Autonomous System Design Through the Lens of Skill-, Rule-, and Knowledge-Based Behaviors", Duke University](https://hal.pratt.duke.edu/sites/hal.pratt.duke.edu/files/u10/IS-29-05-Expert%20Opinion[1]_0.pdf) ] --- name: automation-jobs # Human Supoervisory Control <br> .center[ 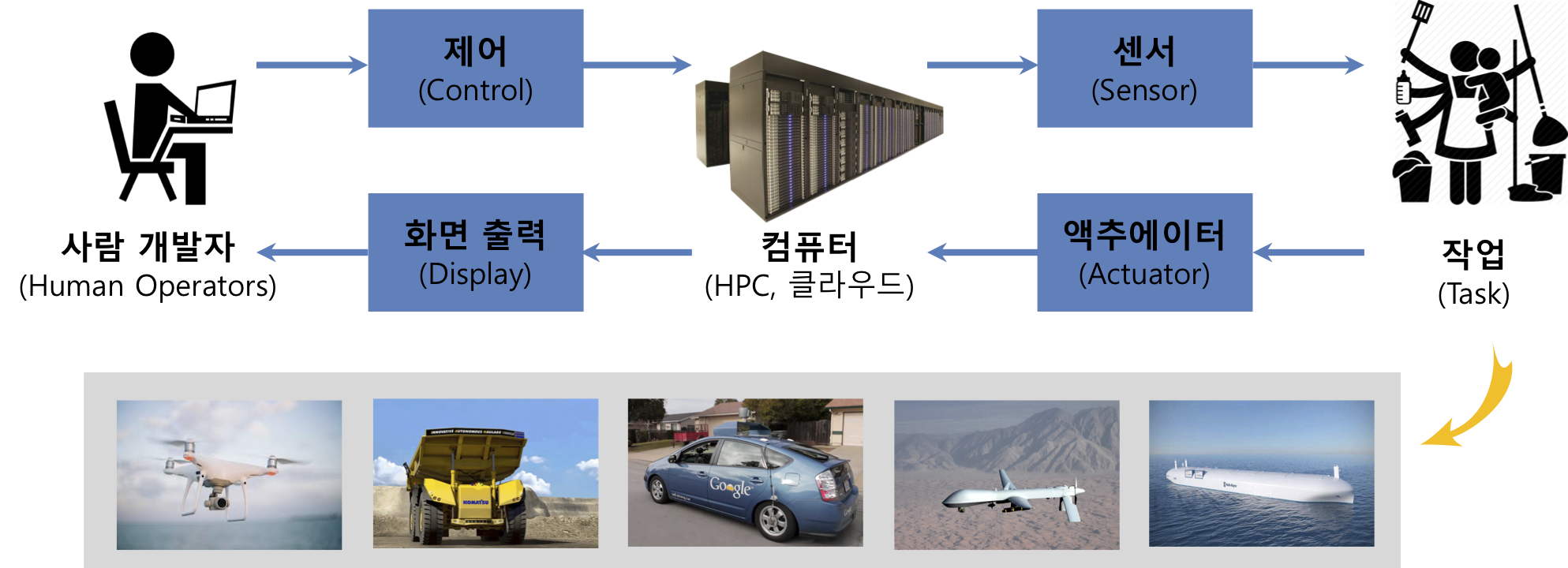 ] .footnote[ [Mary (Missy) Cummings (2017), "Informing Autonomous System Design Through the Lens of Skill-, Rule-, and Knowledge-Based Behaviors", Duke University](https://hal.pratt.duke.edu/sites/hal.pratt.duke.edu/files/u10/IS-29-05-Expert%20Opinion[1]_0.pdf) ] --- name: case-study-document class: inverse, middle # Outline ---- .pull-left[ 경제적인 면에 초점을 두어 .green[**디지털 경제**]의 주요한 동인인 .green[**자동화**]와 .green[**기계학습**]에 대해 살펴봅니다. 개인용 컴퓨터의 보급 이후 만들어진 디지털 유산인 사무 자동화(Office Automation) .warmyellow[**문서**]의 한계와 이를 벗어나려고 하는 다양한 최신 움직임도 짚어봅니다. 디지털 전환의 기본을 이루는 .black[**데이터 과학**]의 핵심적인 내용을 다루고, 다양한 데이터 중 하나인 음성을 텍스트로 변환시킨 후 가치를 창출하는 전반적인 과정을 다뤄보고자 합니다. ] <!--end of left-column--> .pull-right[ .left[ 1\. [자동화(Automation)](#automation) 2\. **[.warmyellow[기계학습(Machine Learning)]](#machine-learning)** 3\. [사무 문서](#case-study-document) 4\. [데이터 과학](#data-science) 5\. [STT](#stt) 6\. [마무리](#goodbye) ] ] <!--end of right-column--> --- name: ml-overview # AI / ML / 통계 <br> .center[ <img src="fig/ai-ml-statistics.png" alt="AI > ML > Statistics" alt="100%" /> ] --- name: ml-sofware # 소프트웨어 1.0과 2.0 - 소프트웨어 1.0: 사람이 직접 규칙(Rule)을 작성하여 똑똑한 시스템 개발 - 소프트웨어 2.0: 데이터에서 딥러닝 알고리즘을 활용 기계가 알고리즘(Algorithm) 제작 .center[ <img src="fig/programming_machine_learning_comparison.png" width="77%"> ] .footnote[ [궁극의 주인이 될 알고리즘(Master Algorithm)](https://statkclee.github.io/ai-lab/master-algorithm.html) ] --- name: ml-bigdata-ml # 빅데이터와 기계학습 .center[ <img src="fig/intree-xy-f.png" alt="정형데이터와 비정형 데이터, 그리고 기계학습" width="90%"> ] .footnote[ - [데이터 가져오기](https://statkclee.github.io/ingest-data/) - [직사각형 데이터](https://statkclee.github.io/data-science/) - [텍스트 데이터](https://statkclee.github.io/text/) - [네트워크 데이터](https://statkclee.github.io/network/) - [지리정보 데이터](https://statkclee.github.io/spatial/) - [이미지 데이터](https://statkclee.github.io/trilobite/) - 오디오/소리 데이터 - ... ] --- name: ml-deep-learning # 딥러닝(Deep Learning) .center[ <img src="fig/three-pillars.png" width="90%"> ] .footnote[ [xwMOOC 딥러닝과 `\(H_2 O\)`](http://statkclee.github.io/deep-learning/) ] --- name: case-study-document class: inverse, middle # Outline ---- .pull-left[ 경제적인 면에 초점을 두어 .green[**디지털 경제**]의 주요한 동인인 .green[**자동화**]와 .green[**기계학습**]에 대해 살펴봅니다. 개인용 컴퓨터의 보급 이후 만들어진 디지털 유산인 사무 자동화(Office Automation) .warmyellow[**문서**]의 한계와 이를 벗어나려고 하는 다양한 최신 움직임도 짚어봅니다. 디지털 전환의 기본을 이루는 .black[**데이터 과학**]의 핵심적인 내용을 다루고, 다양한 데이터 중 하나인 음성을 텍스트로 변환시킨 후 가치를 창출하는 전반적인 과정을 다뤄보고자 합니다. ] <!--end of left-column--> .pull-right[ .left[ 1\. [자동화(Automation)](#automation) 2\. [기계학습(Machine Learning)](#machine-learning) 3\. **[.warmyellow[사무 문서]](#case-study-document)** 4\. [데이터 과학](#data-science) 5\. [STT](#stt) 6\. [마무리](#goodbye) ] ] <!--end of right-column--> <!--------------------------------- 엑셀 -------------------------------------- --> --- name: crisis-excel # 위기의 스프레드쉬트(엑셀) .center[ <img src="fig/train2data-analysis.jpg" alt="분석행" width="67%" /> ] .footnote[ [스터디 뽀개기 7월 (2016-07-30), 분석행(Train to data-analysis)](https://onoffmix.com/event/73626) ] --- name: excel-crisis-accident # 스프레드쉬트 참사 | 회사 | 손실 | 날짜 | 영향 | 참사 개요 | |---------------|-----------|----------|-----------------------|------------------------------------------| | Mouchel | £ 4.3백만 | '10.11월 | CEO 사임, 주가폭락 | 연금펀드평가 £ 4.3백만 엑셀 오류 | | C&C Group | £ 9 백만 | '09.7월 | 주가 15% 하락 등 | 매출 3% 상승이 아니고 5% 하락, 엑셀 오류 | | King 펀드 | £ 130 백만 | '11.05월 | 브래드 이미지 하락 | 웨일즈 지방 NHS 지출 엑셀 오류 | | AXA Rosenberg | £ 150 백만 | '11.02월 | 은폐, 벌금, 브래드 이미지 하락 | 엑셀 오류를 감춰서 $242 백만 벌금 | | JP Morgan Chase | £ 250 백만 | '13.01월 | 명성, 고객 신뢰도 저하 | 바젤 II VaR 위험 평가 엑셀 오류 | | Magellan 펀드 | £ 1.6 십억 | '95.01월 | 투자자에게 약속한 배당금 지급 못함 | 음수 부호 누락으로 자본이득 과대계상| | 미연방준비위원회 | £ 2.5 십억 | '10.10월 | 명확하지 않음 | 리볼빙 카드 신용액 산출 과정에 엑셀 오류 | .footnote[ [THE DIRTY DOZEN 12 MODELLING HORROR STORIES & SPREADSHEET DISASTERS](http://blogs.mazars.com/the-model-auditor/files/2014/01/12-Modelling-Horror-Stories-and-Spreadsheet-Disasters-Mazars-UK.pdf) ] --- name: why-leave-excel # 스프레드쉬트를 버려야 하는 6가지 이유 ### 스프레드쉬트를 사용하는 이유 > > * 항상 엑셀을 사용했다: 항상 데이터를 엑셀로 저장하고 분석해서 사용했다. 하지만, 버튼 하나로 데이터를 내보내기 쉽다. > * 데이터베이스를 사용할 만큼 데이터가 충분하지 않다: 데이터가 작아서 데이터베이스를 사용할 이유를 찾지 못하지만, 사업이 커지고, 업무량이 늘어나면서 엑셀 작업량이 높아지고, 복잡성도 커지고, 엑셀 자체에서 처리되는 시간도 늘어난다. ### 스프레드쉬트를 떠나야하는 이유 1. 한번에 한사람만 작업이 가능하다. 1. 데이터 감사는 데이터베이스에서만 가능 1. 데이터베이스는 정형화된 작업흐름을 지원한다. 1. 데이터베이스는 엑셀보다 모형을 보다 잘 지원한다. 1. 데이터베이스로 보고서 생성이 수월하다. 1. 데이터베이스는 보안이 강력하고 규제하기 좋다. .footnote[ [데이터 과학, "위기의 스프레드쉬트"](https://statkclee.github.io/data-science/ds-why-not-excel.html) ] --- name: excel-complexity # 스프레드쉬트 알고리즘 복잡성 <br> <br> <br> .center[ <img src="fig/ds-xls-algo-complexity.png" alt="알고리즘 복잡성" width="100%" /> ] .footnote[ [탈옥(jailbreakr) – 엑셀에서 탈출… 자유](https://statkclee.github.io/data-science/ds-xls.html) ] --- name: reason-excel-complex # 스프레드쉬트 복잡성과 효율성 <br> <br> .pull-left[ - 스프레드쉬트는 데이터, 서식, 수식으로 구성된다. - 숫자 데이터를 엑셀로 가져오게 되면 엑셀 내장 함수를 통해 수식 계산을 수행한다. - 엑셀 사용자 본인 혹은 외부 사람을 위해 서식을 입히는 과정을 거쳐 비로소 완성된 스프레드쉬트가 된다. ] .pull-right[ .center[ <img src="fig/ds-xls-data-format-logic.png" alt="알고리즘 복잡성" width="100%" /> ] ] .footnote[ [탈옥(jailbreakr) – 엑셀에서 탈출… 자유](https://statkclee.github.io/data-science/ds-xls.html) ] <!--------------------------------- 파워포인트 -------------------------------------- --> --- name: crisis-powerpoint # 파워포인트의 종말 ← 아마존 .center[ <img src="fig/no-powerpoint.jpeg" alt="노 파워포인트" width="57%" /> ] .footnote[ [김미리 기자 (2019.04.06.), "보고서 치장은 그만, 글로 써라.. '제로 PPT' 선언하는 기업들", 조선일보](https://news.v.daum.net/v/20190406030158105) ] --- name: three-paradigms # 세가지 다른 글쓰기 패러다임 .center[ <img src="img/data-conference-three-languages.png" alt="세가지 패러다임" width="120%"/> ] .footnote[ [심각한 현재 상황](http://statkclee.github.io/modern-scientific-authoring/01-mess-kr.html) ] --- name: automation ## 도구가 자동화하는 저작 업무 .pull-left[ ### 1. `\(LaTex\)` 사례 <img src="fig/latex-how-it-works.png" alt="도구가 자동화하는 저작 업무" width="100%" /> [정보교육을 위한 파이썬: 데이터 과학자로의 여정, 아마존 절찬 판매중](https://statkclee.github.io/pythonlearn-kr/01-py4inf-python2/) ] .pull-right[ ### 2. 북다운(Bookdown) .center[ <img src="fig/00_cover_boy_girl.png" alt="컴퓨터 과학 언플러그드" width="100%" /> ] [컴퓨터 과학 언플러그드, 이광춘 번역](https://statkclee.github.io/unplugged/) ] --- name: automation-continued ## 도구가 자동화하는 저작 업무 .pull-left[ ### 3. SW 도움말 <img src="fig/latex-sphinx.png" alt="파이썬 스핑크스 저작 흐름" width="100%" /> - [Welcome to Reeborg world!](http://reeborg.ca/index_en.html) - [리보그 한국어 버젼](http://reeborg.ca/docs/ko/index.html) - [프로그래밍과 문제해결: 파이썬, 리보그, 러플](https://statkclee.github.io/code-perspectives/) ] .pull-right[ ### 4. 블로그 .center[ <img src="fig/blogdown-deployment.png" alt="정적 웹콘텐츠 배포" width="100%" /> ] [데이터 과학을 위한 저작도구 블로그 - `blogdown`](https://statkclee.github.io/ds-authoring/ds-blogdown.html) ] [xwMOOC 블로그 - `netlify`](http://xwmooc.netlify.com/) --- name: automation-slideshow ## 도구가 자동화하는 저작 업무 ### 5. 슬라이드쇼(slideshow) .center[ <img src="fig/slideshow.png" alt="슬라이드쇼" width="50%" /> ] .footnote[ [소프트웨어에 물들다(왕곡초등학교, 2018-05-26)](somul_20180525.html) - 글쓰기를 잘하기 위해서 알아야 되는 코딩 ] --- name: slideshow-final-boss # 파워포인트 → AI .center[ <iframe src="https://statkclee.github.io/ds-authoring/seoul_r_xaringan.html#1" width="100%" height="400px"></iframe> ] .footnote[ [이광춘 (2020-12-12), "파워포인트를 넘어서 - xaringan", Seoul R Meetup 2020](https://tidyverse-korea.github.io/seoul-R/) ] <!--------------------------------- 워드 -------------------------------------- --> --- name: slideshow-final-boss # 아래한글, 워드 → computational documents .pull-left[ <iframe src="https://statkclee.github.io/ds-authoring/KCD2020_Tidyverse.html#1" width="100%" height="400px"></iframe> ] .pull-right[ <iframe width="560" height="315" src="https://www.youtube.com/embed/R5nD-VuzzD8" frameborder="0" allow="accelerometer; autoplay; clipboard-write; encrypted-media; gyroscope; picture-in-picture" allowfullscreen></iframe> ] .footnote[ [이광춘 (2020-11-07), "코딩으로 글쓰는 슬기로운 탐구생활 발표자료", KCD(Korea Community Day) 2020](https://tidyverse-korea.github.io/seoul-R/) ] --- name: case-study-document class: inverse, middle # Outline ---- .pull-left[ 경제적인 면에 초점을 두어 .green[**디지털 경제**]의 주요한 동인인 .green[**자동화**]와 .green[**기계학습**]에 대해 살펴봅니다. 개인용 컴퓨터의 보급 이후 만들어진 디지털 유산인 사무 자동화(Office Automation) .warmyellow[**문서**]의 한계와 이를 벗어나려고 하는 다양한 최신 움직임도 짚어봅니다. 디지털 전환의 기본을 이루는 .black[**데이터 과학**]의 핵심적인 내용을 다루고, 다양한 데이터 중 하나인 음성을 텍스트로 변환시킨 후 가치를 창출하는 전반적인 과정을 다뤄보고자 합니다. ] <!--end of left-column--> .pull-right[ .left[ 1\. [자동화(Automation)](#automation) 2\. [기계학습(Machine Learning)](#machine-learning) 3\. [사무 문서](#case-study-document) 4\. **[.warmyellow[데이터 과학]](#data-science)** 5\. [STT](#stt) 6\. [마무리](#goodbye) ] ] <!--end of right-column--> --- name: data-science # 데이터 과학 데이터 사이언스(Data Science)는 데이터를 다루는 과학이다. 따라서 자연법칙을 다루는 자연과학과 맥이 닿아있다. 자연법칙을 측정하여 데이터프레임(`DataFrame`)으로 나타내면 **변수(Variable)**, **관측점(Observation)**, **값(Value)**으로 표현된다. <img src="fig/data-science-overview.png" alt="데이터 사이언스 개요" width="100%" /> .footnote[ [Google Search - "데이터 과학"](https://www.google.com/search?q=%EB%8D%B0%EC%9D%B4%ED%84%B0+%EA%B3%BC%ED%95%99) ] --- name: tidyverse # AI/ML/DL vs 데이터 과학 <br> .center[ <img src="fig/tidyvese_os.png" width="77%" /> ] .footnote[ [한국통계학회 소식지 2019년 10월호 ](https://statkclee.github.io/ds-authoring/ds-stat-tidyverse.html) ] --- name: tidyverse-definition # `tidyverse` <br> .center[ <img src="fig/maso_tidyverse.png" width="77%" /> ] .pull-left[ - 기존 자료구조를 재사용 - 파이프 연산자로 간단한 함수를 조합 - 함수형 프로그래밍을 적극 사용 - 기계가 아닌 인간을 위한 설계 ] .pull-right[ - Reuse existing data structures. - Compose simple functions with the pipe. - Embrace functional programming. - Design for humans. ] --- name: y-fx # 30년의 변화를 그림으로 표현 .center[ <img src="fig/intree-xy-f.png" alt="XY" width="77%" /> ] .footnote[ [이광춘(2019-01-26), "Data Scientist 가 바라본 제조업의 발전 전략 및 방안", Intree 4.0 World Forum](https://statkclee.github.io/ds-authoring/intree-seminar-2019-01.html) ] --- name: data-science-graph ## Big Data/AI/ML/Data Science <br> .pull-left[ ### Google Trends: 미국 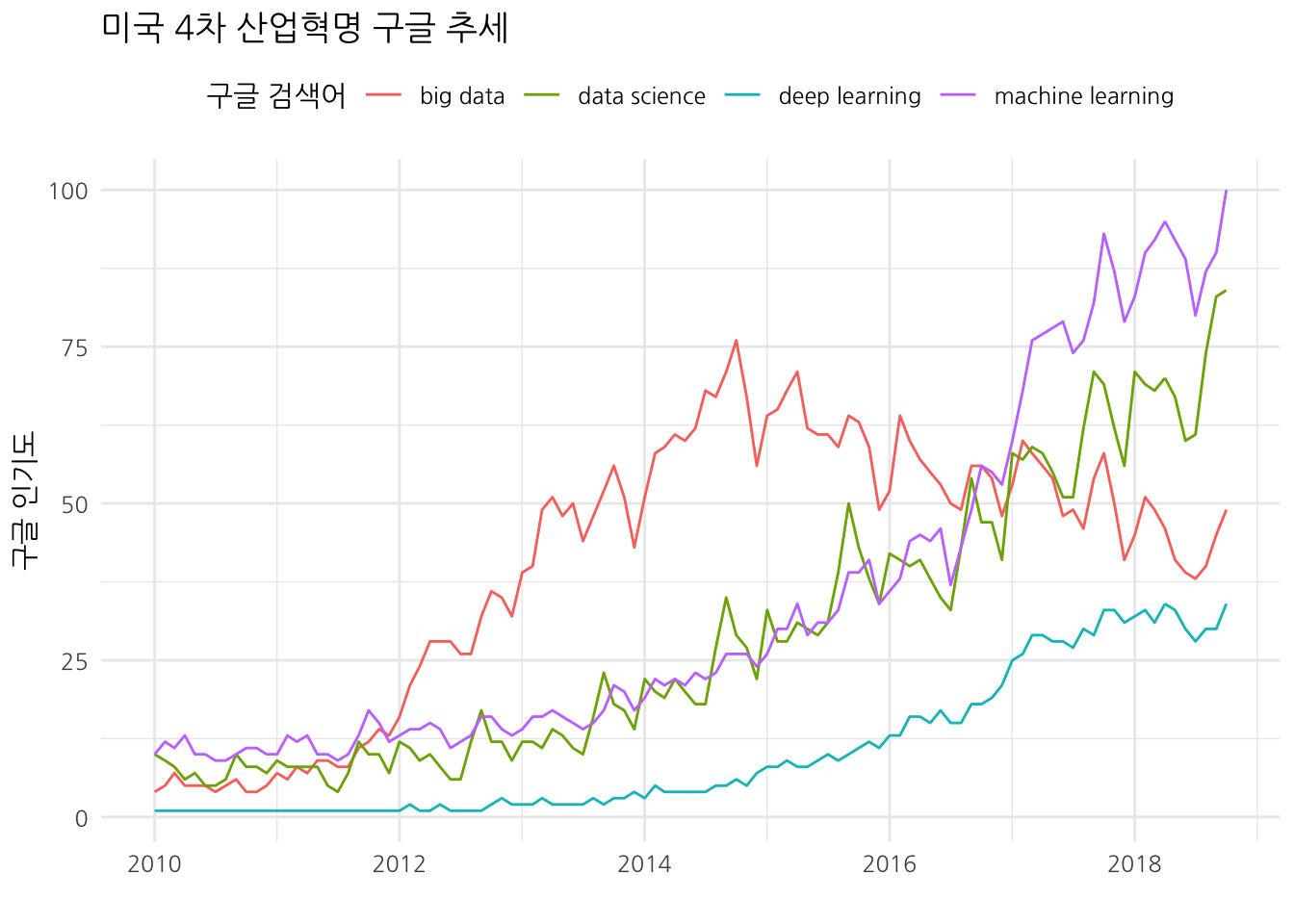 ] .pull-right[ ### Google Trends: 대한민국 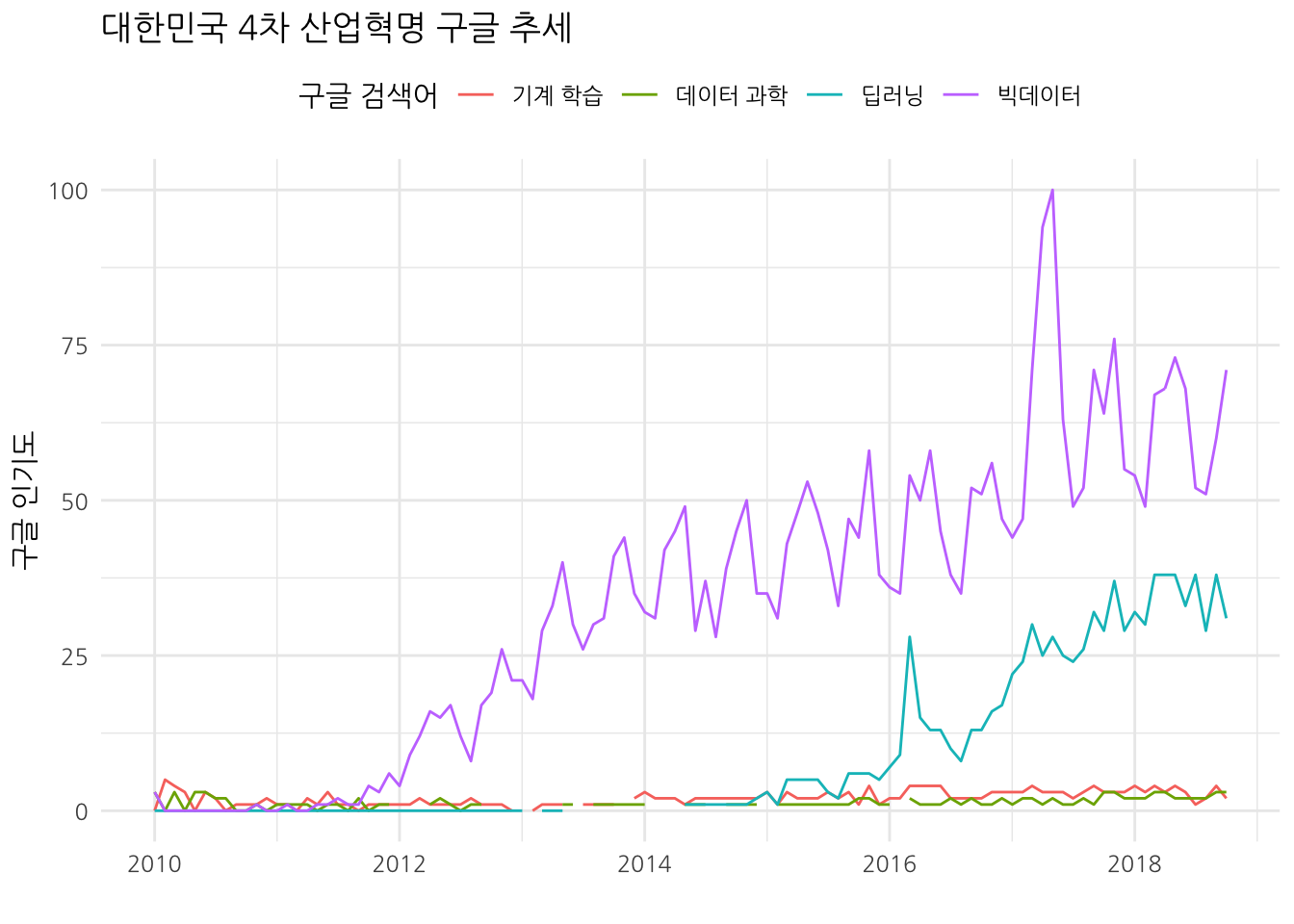 ] .footnote[ [이광춘 (2020-07-21), "기업운영혁신을 위한 데이터 과학: 기업의 활용방안", 포항산업과학연구원(RIST)](https://statkclee.github.io/ds-authoring/ds-rist.html) ] --- name: data-science-resource # 데이터 경제와 산업 .pull-left[ ### 데이터 자원 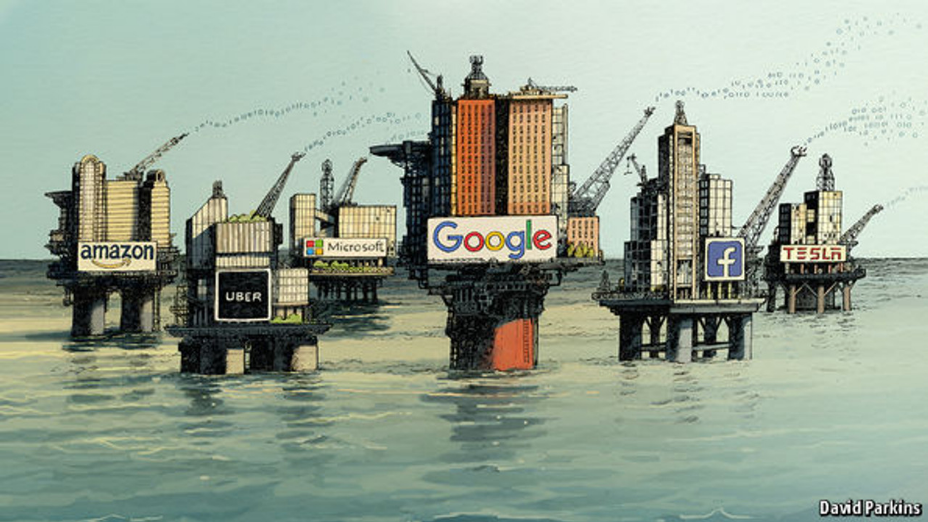 ] .pull-right[ ### 파이프라인 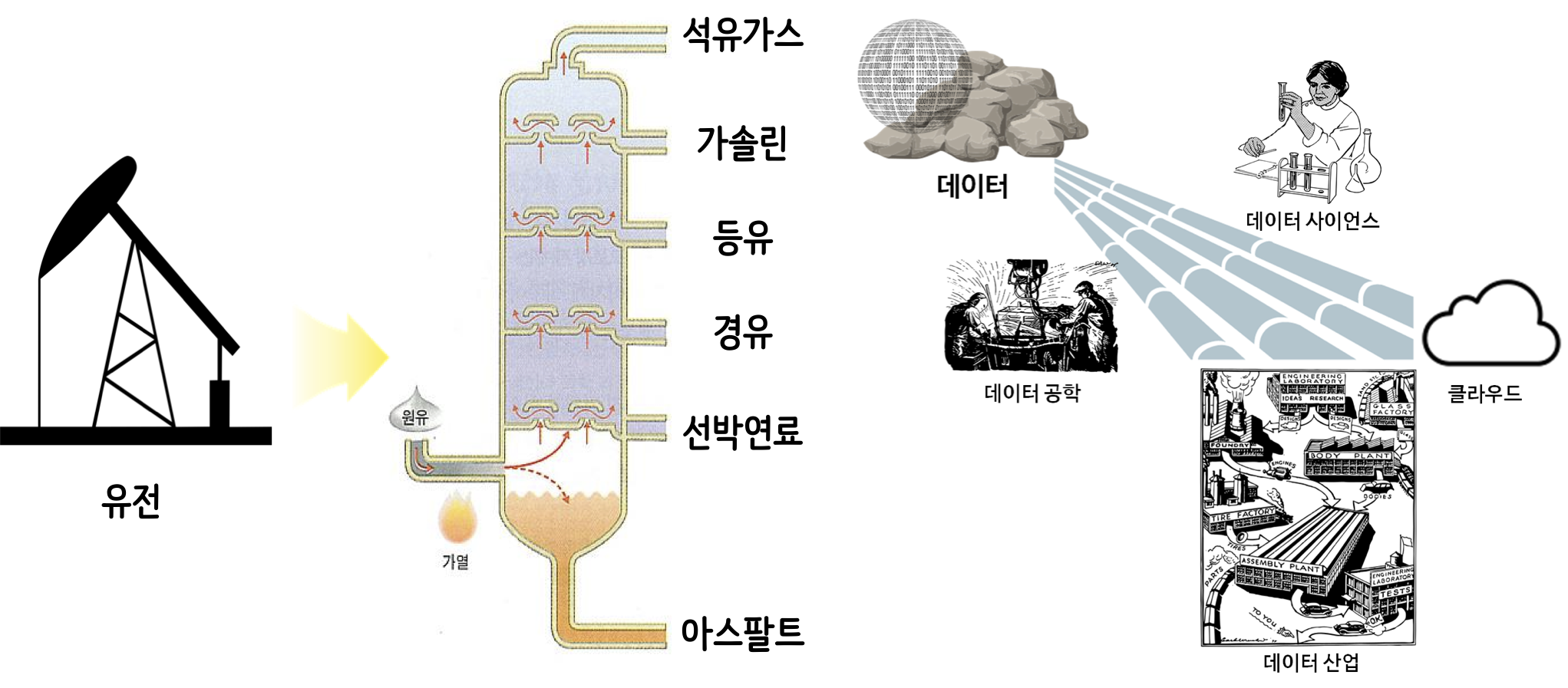 ] .footnote[ [이광춘 (2020-07-21), "기업운영혁신을 위한 데이터 과학: 기업의 활용방안", 포항산업과학연구원(RIST)](https://statkclee.github.io/ds-authoring/ds-rist.html) ] --- name: data-sciece-value ## 가치 <small> <ul> <li>기술 분석(Descriptive Analytics): 무슨 일이 있었나? (What happened?)</li> <li>진단 분석(Diagnostic Analytics): 왜 일어났나? (Why did it happen?)</li> <li>예측 분석(Predictive Analytics): 무슨 일이 일어날까? (What will happen?)</li> <li>처방 분석(Prescriptive Analytics): 어떻게 그런 일을 일으킬까? (How can we make it happen?)</li> </ul> </small> .center[ <img src="fig/ds-gartner.png" width = "80%" /> ] --- name: data-sciece-cost # 패러다임의 변화 .pull-left[ ### 비용 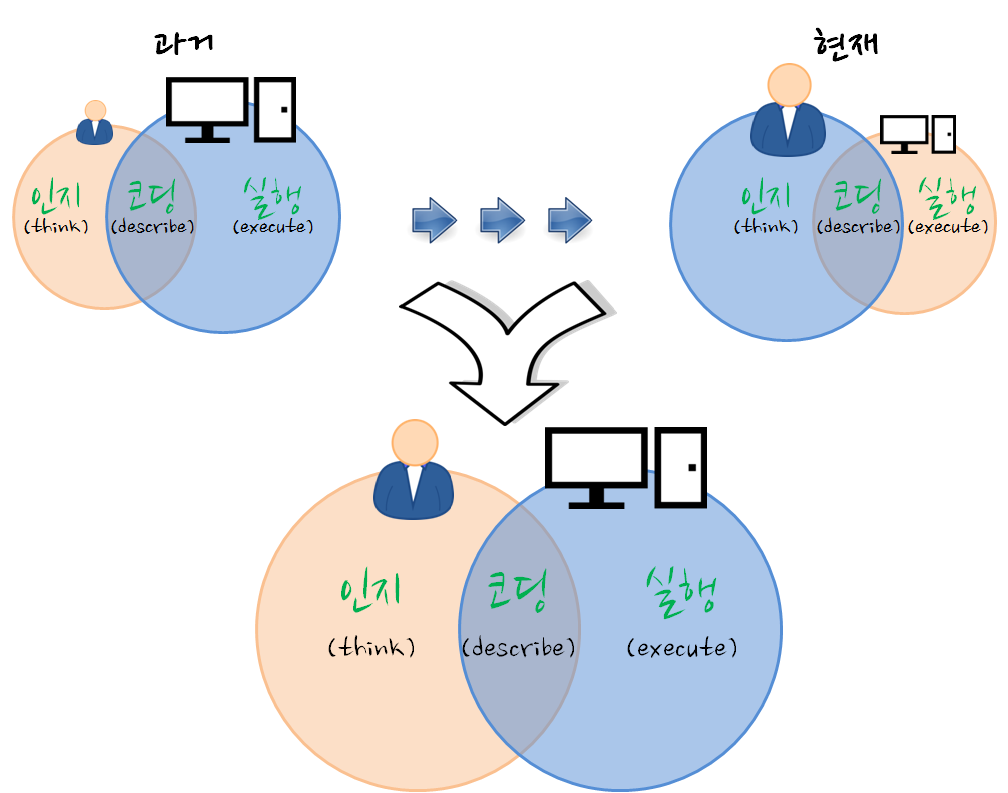 ] .pull-right[ ### 자동화 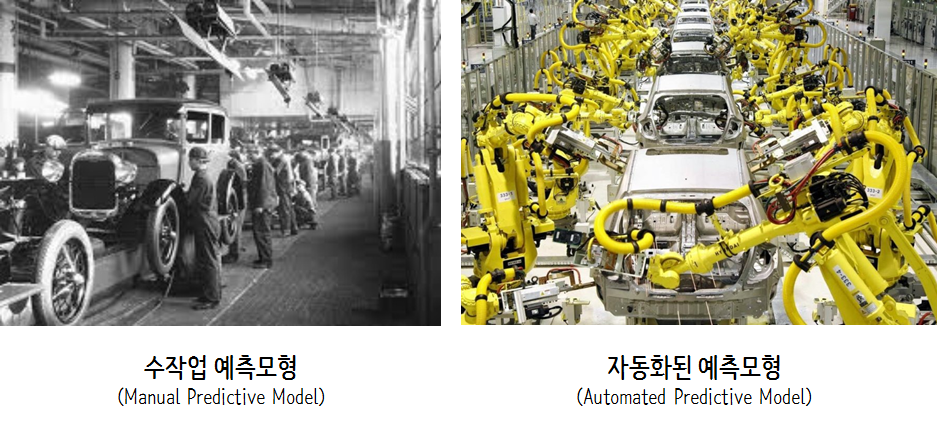 ] --- name: case-study-document class: inverse, middle # Outline ---- .pull-left[ 경제적인 면에 초점을 두어 .green[**디지털 경제**]의 주요한 동인인 .green[**자동화**]와 .green[**기계학습**]에 대해 살펴봅니다. 개인용 컴퓨터의 보급 이후 만들어진 디지털 유산인 사무 자동화(Office Automation) .warmyellow[**문서**]의 한계와 이를 벗어나려고 하는 다양한 최신 움직임도 짚어봅니다. 디지털 전환의 기본을 이루는 .black[**데이터 과학**]의 핵심적인 내용을 다루고, 다양한 데이터 중 하나인 음성을 텍스트로 변환시킨 후 가치를 창출하는 전반적인 과정을 다뤄보고자 합니다. ] <!--end of left-column--> .pull-right[ .left[ 1\. [자동화(Automation)](#automation) 2\. [기계학습(Machine Learning)](#machine-learning) 3\. [사무 문서](#case-study-document) 4\. [데이터 과학](#data-science) 5\. **[.warmyellow[STT]](#stt)** 6\. [마무리](#goodbye) ] ] <!--end of right-column--> --- name: ml-overview # STT 사례 <br> .center[ <img src="fig/stt-google.png" alt="" alt="100%" /> ] .footnote[ [speech-to-text - 음성을 텍스트로 변환](https://statkclee.github.io/deep-learning/r-stt.html) ] --- name: ml-overview # 자연어 처리 흐름 <br> .center[ <img src="fig/text-mining-workflow.png" alt="" alt="100%" /> ] .footnote[ [텍스트 데이터](https://statkclee.github.io/text/nlp-text.html) ] --- name: ml-overview # 언어기술 로드맵 <br> .center[ <img src="fig/ml-text-technology-roadmap.png" alt="" alt="100%" /> ] .footnote[ [단어주머니(Bag of Words)](https://statkclee.github.io/text/nlp-bag-of-words.html) ] --- name: goodbye class: middle, inverse .pull-left[ # **경청해 주셔서 <br>감사합니다.** <br/> ## Tidyverse Korea ] .pull-right[ .right[ <img style="border-radius: 100%;" src="../fig/Tidyverse_Korea_logo_transparent.png" width="300px"/> ] ]